Jul 27: Automated surveillance of healthcare-associated infections
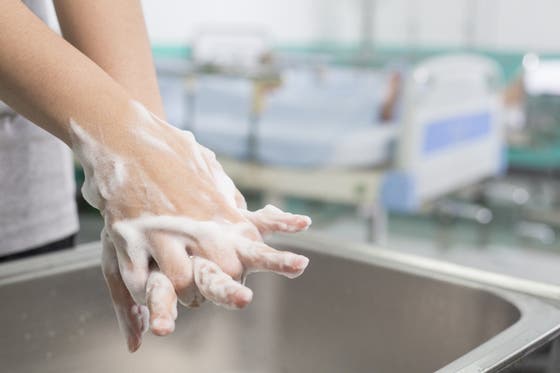
Automated surveillance of healthcare-associated infections uses routine-care data stored in electronic health records (EHR) to identify patients who (may) have developed a HAI. These systems reduce the workload of manual surveillance, thereby freeing up infection control practitioners' time; in addition, it can provide better standardization of surveillance results by facilitating data collection and standardizing case ascertainment.
A guidance document, written by the PRAISE network (Providing a Roadmap for Automated Infection Surveillance in Europe) under responsibility of Maaike van Mourik, PhD (Department of Medical Microbiology, UMC Utrecht) on automated surveillance was recently published as a supplement in Clinical Microbiology & Infection.
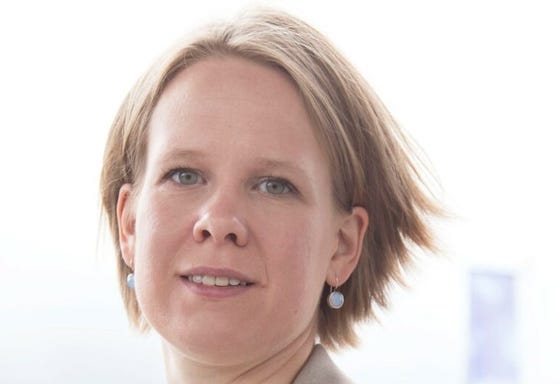
Maaike van Mourik, PhD
Healthcare-associated infections (HAI) such as surgical site infections and central line–associated bloodstream infections are among the commonest adverse events of medical care and are estimated to affect 6.5 percent of patients in acute care hospitals on any given day. Surveillance of HAI and feedback of their rates, in particular within surveillance networks, is a key component of successful infection prevention programs that provides caregivers and policy makers with the necessary information to identify areas of improvement and guide interventions.
Surveillance of healthcare-associated infections
Conventional surveillance of HAI is done by manually reviewing patients' medical records and ascertaining the presence of HAI according to standardized surveillance definitions. This is a time-consuming and resource-intensive effort, and there are concerns regarding the uniformity of the surveillance results. These shortcomings of conventional HAI surveillance have led to the development and use of automated surveillance (AS) systems that use routine-care data stored in electronic health records (EHR) to identify patients who (may) have developed a HAI. These systems reduce the workload of manual surveillance, thereby freeing up infection control practitioners' time; in addition, it can provide better standardization of surveillance results by facilitating data collection and standardizing case ascertainment. These benefits of AS, combined with the increasing availability of data stored in EHR and the need for large-scale surveillance data, could motivate many hospitals, public health institutes and surveillance networks to transition to automated surveillance.
However, this transition is not without risk. Guidance is lacking on how to best automate the surveillance process and ensure the delivery of surveillance data that is uniform and useful for improving the quality of care. In addition, the transition to automated surveillance entails more than converting a manual process to an automated process; it will affect surveillance targets, definitions, methods and interpretation of data, and it thereby runs the risk of losing clinical buy-in. AS has been applied in the research setting within hospitals and sparsely in the setting of large-scale AS. However, many of the currently available AS systems were developed in individual institutions with specific local preferences and conditions, and hence they are diverse in their aims, definitions, design and methods used. This solitary, stand-alone development of AS systems leads to heterogeneity and poor interoperability between systems. In addition, not all healthcare facilities can make a transition to AS on their own, leading to a segregation in healthcare facilities that collect surveillance data manually and automatically. These factors jeopardize the possibility of using surveillance data for comparison and subsequent quality improvement.
PRAISE Network
The PRAISE network (Providing a Roadmap for Automated Infection Surveillance in Europe) was initiated in 2019 to develop guidance support the transition to large-scale AS and recently published a roadmap that offers high-level conceptual guidance for the development of surveillance systems. It pays extensive attention to the selection of surveillance targets and definition and the design of AS systems (including selection of data sources and algorithms), and it discusses implementation considerations, validation, maintenance and areas of future research. The roadmap is accompanied by an article on the information technology (IT) requirements of large-scale AS, including the (re)use of healthcare data from EHR, standardization, interoperability, IT architecture and secure data transfer. The supplement is completed by and article discussing governance aspects that are of particular importance in large-scale AS systems, including engagement of stakeholders, transparency of algorithms and accountability and the legal and ethical principles regarding the reuse of personal data for the purpose of surveillance.
Publication
Mourik M van (Editor). Implementing Automated Surveillance of Healthcare-Associated Infections (HAI). Clin Microbiol Infect 2021;27(Suppl. 1):S1-S40